2022 - TBD (PhD Research)
Autumn 2022 - From the Ashes
The eruption of Mount St. Helens in 1980 resulted in a cataclysmic restructuring of its surrounding landscapes. The Pumice Plain is one of these landscapes, where tree species such as Sitka willow (Salix sitchensis) and their dependent communities have established along newly-formed streams. Thus, the study of these dependent communities provides a unique and rare opportunity to investigate factors influencing metacommunity assembly during true primary succession. We analyzed the influence of landscape connectivity on metacommunity assembly through a novel application of circuit theory, alongside the effects of other factors such as stream locations, willow leaf chemistry, and leaf area. We found that landscape connectivity structures community composition on willows across the Pumice Plain, where the least connected willows favored active flyers such as the western tent caterpillar (Malacosoma fragilis) or the Pacific willow leaf beetle (Pyrrhalta decora carbo). We also found that multiple levels of spatial habitat structure linked via landscape connectivity can predict the presence of organisms lacking high rates of dispersal, such as the invasive stem-boring poplar weevil (Cryptorhynchus lapathi). This is critical for management as we show that the maintenance of a heterogeneous mixture of landscape connectivity and resource locations can facilitate metacommunity dynamics to promote ecosystem function and mitigate the influences of invasive species.
Spring 2022 - The Grass is Always Greener
Salt marshes are globally important ecosystems but due to the impacts of global change, many of these ecosystems have been lost or transformed. There have been previous attempts to broadly quantify salt marsh communities, specifically the ubiquitous grasses which serve as foundation species (Spartina alterniflora and Spartina patens), the latter of which being less tolerant of sea-level rise. However, few researchers have used high-resolution geospatial imagery to quantify fine-scale changes in the distribution of grasses. Thus, we developed a novel, easy-to-use method of classifying geospatial data with supervised machine learning that enables a user to iteratively build datasets based on their own ecological knowledge. Our methods allowed us to characterize immense regions (two geospatially proximal areas, >7,000 ha each) of critical salt marshes on the New Jersey coast and to evaluate fine-scale (1m × 1m) community transformations in response to global change using several years of historical imagery. We achieved strikingly high classification accuracy for all years, with upwards of 98.75% correct for our final model in 2019, and up to 100% of our main species of interest, S. patens. These results confirm the viability of our classification methods for broad-scale community characterization. Analyzing classifications from 2006 to 2019, we found vastly different patterns of community response in our target marshes. One marsh appeared to experience very little change while the other experienced an incredible 81.17% (1,087 ha) reduction of S. patens. These results signify the importance for future broad-scale ecological studies to not generalize across landscapes of an ecosystem-type. Our methods can thus be used to capture the full range of variation in global change responses.
2019-2021 (MS Research) - We Didn't Start the Fire
The evaluation of fire behavior prediction models is an important step forward in understanding the appropriate tool to use in a given ecosystem or climate. Furthermore, the generation of a robust set of fire behavior data across comprehensive fuel structures and fire weather allows for a deeper understanding of how ecology and climate impact fire behavior prediction. When these evaluations are combined, inferences and suggestions can be made that have the power to aid future research and management decisions. The results of this project are intended to provide guidance for future research and management within the moorlands and heathlands of the UK and Norway. Further, it is hoped that the James Hutton Institute of Dundee, Scotland, will be able to compare their characterization of vegetation and fire weather with this project’s prediction of fire behavior to aid in the creation of Scotland’s fire danger rating system.
Key findings from this project indicate that the Rothermel model provides an accurate representation of fire behavior for oceanic heathlands and moorlands, as compared to their ecosystem-specific empirical models. This is important as the Rothermel model allows a user to alter fuel input chemistry and physics which makes it potentially more apt to predict fire behavior across a wider range of fuel structures within the same ecosystem. Further, fire behavior predictions across all generated landscapes, and across all fire weather scenarios, indicate that the lowest risks for adverse fire behavior exist in landscapes with the greatest heterogeneity (e.g., landscapes which experience fuel management, typically in the form of managed burning). This means that current attempts to reforest, or “rewild,” landscapes that occur across UK heathlands, moorlands, and peatlands could result in an increase in short-term wildland fire risk.
The management of UK and Norwegian heathlands and moorlands for livestock grazing is an important land-use that was not considered for the purposes of this study which overlaps fire management in many areas. Vandvik et al. found that grazing and fire do not have additive effects on community diversity, rather that the combination provided ecosystems with a complex range of disturbances that created a large amount of niche variance. For British heathalnds, Bullock and Pakeman found that introduction of grazing on Calluna increased plant and bryophyte species richness and relative covers while reducing the amount of highly flammable dead fuels. These findings, in conjunction with the lower diversity of mosses and plant species richness found in birch forests, exemplify the necessity to preserve current management practices that combine the disturbances of grazing and managed burning.
In the outset of this project, there was an intense learning curve to properly implement various different types of fire behavior prediction models. The alignment of these models, in many cases, was a huge success. Further, this project was originally intended to encompass data collection in oceanic heathlands for the purposes of fire behavior modeling. Global occurrences during the breadth of this project, however, prevented traveling to foreign countries. Another important success of this project involved the assimilation of fuels data into characteristics useable by the Rothermel model, especially when considering differences in the way fuel data were collected across different projects. This process was incredibly time-consuming and intensive, involving a detailed and comprehensive knowledge of varying fuel data samples. Other limitations of this project included the simplistic nature of fire behavior prediction using the R ‘Rothermel’ package where only rates of spread could be produced. Other measures of fire behavior are often used to describe impacts on ecosystems, such as fireline intensity, which would have been beneficial to report on in this study.
The results of this project provide a baseline for an array of potential future work. For example, more work needs to be done to understand whether the Rothermel model tolerably predicts fire behavior in other novel ecosystems of the world, not just in the UK and Norway’s oceanic climates. More work should also be done that aligns transitions of Calluna-dominated landscapes with areas of birch forestland, so that the assertions made by this study may either be corroborated or rejected. Further, any future research should aim to collect fuels in-field for the purposes of expanding the fire behavior predictions this study provides for oceanic climates.
Summer 2018 - Bogged Down
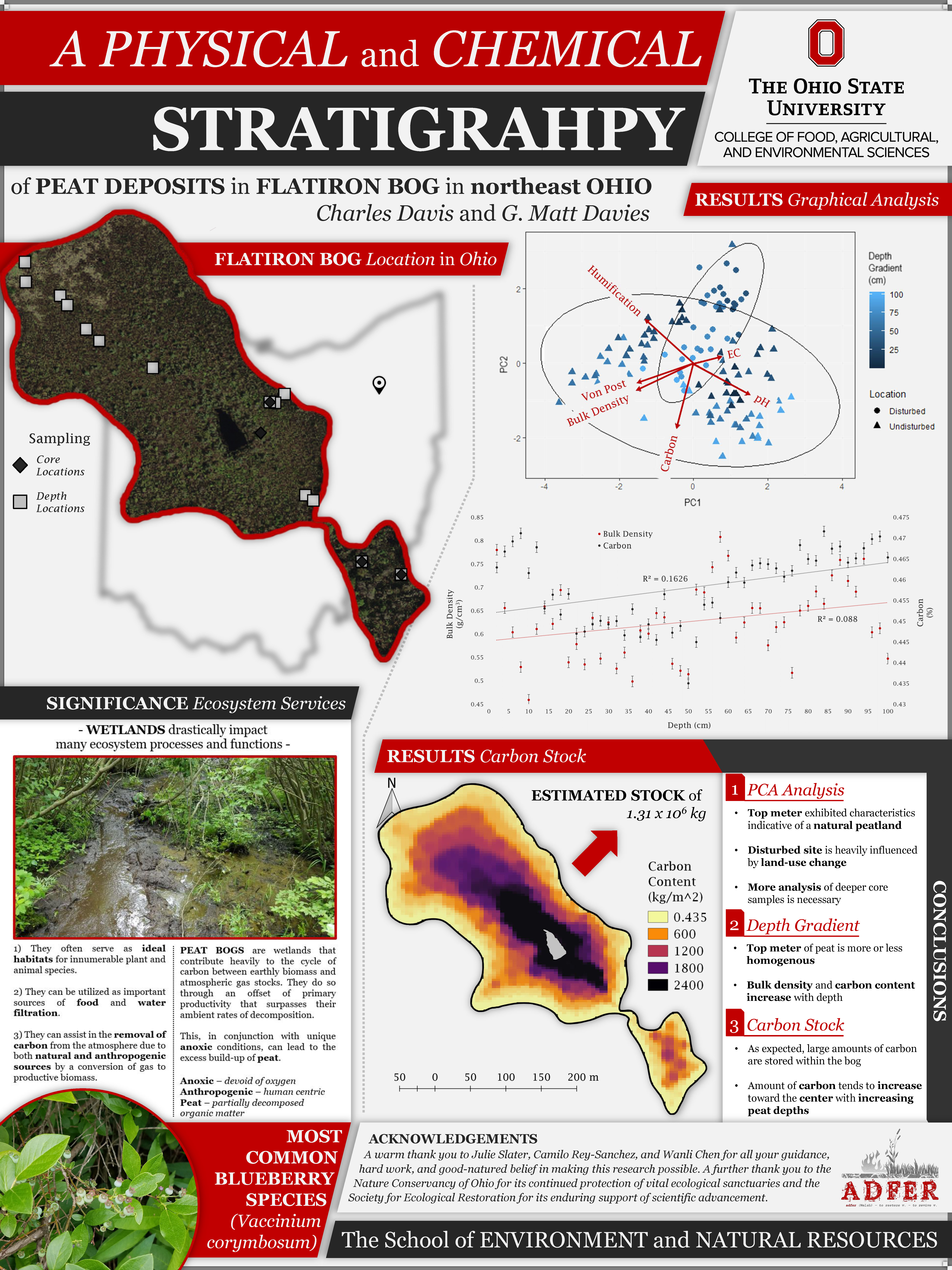